Data Privacy with Privacy Enhancing Technologies: A Guide by ICO and DSIT
- Katarzyna Celińska
- Nov 21, 2024
- 2 min read
In a world where privacy and data security are paramount, the UK Information Commissioner’s Office (#ICO), alongside the Department for Science, Innovation, and Technology (#DSIT), has released a comprehensive Cost-Benefit Awareness Tool to guide organizations on the deployment of Privacy Enhancing Technologies (#PET). PETs enable organizations to process sensitive information securely while mitigating the risks of data breaches and misuse, making them essential for privacy-conscious organizations across sectors.
What Are PETs?
PETs encompass a broad range of technologies designed to safeguard privacy, including:
- Homomorphic Encryption (HE): Allows computation on encrypted data without decryption.
- Trusted Execution Environments: Creates isolated secure areas within processors.
- Secure Multi-Party Computation: Enables collaborative data processing without revealing sensitive details.
- Synthetic Data: Artificially generated datasets preserving the statistical patterns of the original.
- Differential Privacy: Adds random noise to protect data during analysis.
These technologies ensure data privacy while enabling innovations in data-driven decision-making, federated learning, and secure data sharing.
Key Considerations for Deploying PETs
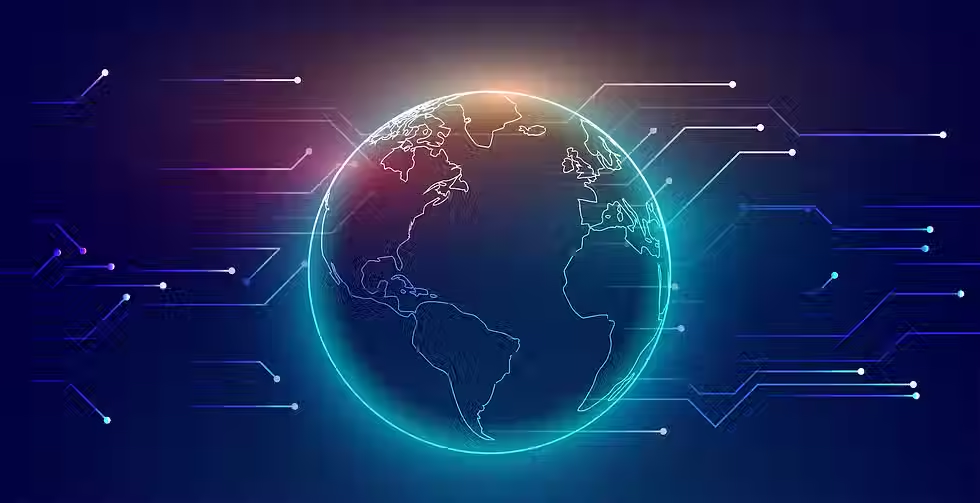
Before implementing PETs, organizations should:
Understand Costs and Benefits: Assess the trade-offs between privacy and utility. For instance:
- HE provides maximum security but involves high computational costs.
- Synthetic data reduces privacy risks but may compromise data utility.
Ensure Legal Compliance: Use PETs to align with GDPR and other data protection laws, reducing liability from data breaches.
Evaluate Infrastructure Needs: PETs may require advanced hardware (e.g., for TEEs) or specialized skills to deploy and maintain.
Prepare for Operational Challenges: Debugging and testing PET-based systems can be complex, requiring robust troubleshooting pathways.
Use Cases
- Healthcare: Federated learning enables cross-border collaboration on disease analysis without sharing patient data.
- Finance: SMPC allows organizations to compute shared metrics like average salaries without exposing individual data.
- AI Development: Synthetic data supports AI training without compromising real user data.
Autor: Sebastian Burgemejster
Comments